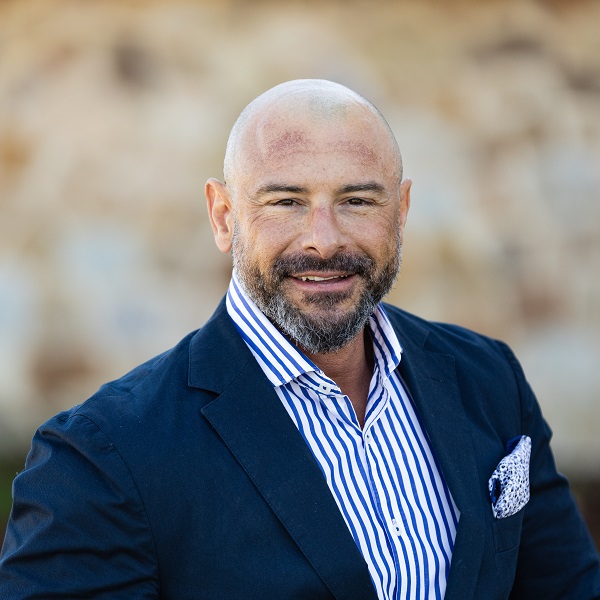
The next horizon with GenAI is competitive differentiation
By George Dragatsis (pictured), ANZ Chief Technology Officer, Hitachi Vantara
By now, most organisations have dipped into GenAI in some form. Its transformative power as a technology is well understood, and efforts to date have proven that value from using it can be realised.
What’s interesting, though, is how organisations have embraced GenAI to date, how that’s now in the process of changing, and what that means from an investment and data management perspective.
A recent study from Hitachi Vantara based on a survey by the Enterprise Strategy Group (ESG) uncovered an interesting trend: that to date, organisations have taken a fairly generic and affordable approach to GenAI.
Some of this is to be expected: most organisations were introduced to GenAI through ChatGPT, a publicly-accessible and low-cost service.
While security and risk concerns may have persuaded some enterprises to shift to alternative tooling, the ESG-backed survey found most organisations have not strayed too far from accessible and non customised models. About 65% of respondents have gone with “commercial and off-the-shelf”, “off-the-shelf open source” or “free and off-the-shelf” options to get started with GenAI. Another 31 percent are backing an open source option with an unspecified amount of customisation.
The flipside of that is that organisations have so far shunned the use of more proprietary – read internally-developed – large language models (LLMs), though this is set to change. Use of proprietary models is expected to increase in the long term as organisations prioritise competitive differentiation as a key outcome of their GenAI efforts.
One of the challenges associated with the current reliance on low-cost, generic models is that the outputs are largely untailored to the user organisation. Any organisation that makes use of the model can likely achieve close to the same result. That may be fine if the goal is simply to experiment or to prove out a business case, but the effort invested runs the risk of going largely unrewarded, since every organisation can achieve the same level of uplift if they so choose. Everyone wins, but also no one wins.
Where organisations want to get to with GenAI, as with any investment in technology, is a point where it allows them to create or foster a real competitive advantage – where what they do has a material and measurable impact on transactional or business efficiency, and/or on the business bottom line.
The power play of in-house development and internal data
The Hitachi-ESG study maps out the changing mix of LLMs in use as competitive advantage outcomes are prioritised in GenAI programmes and efforts.
A key trend is that a blend of models – or at least of deployment options – is likely to be favoured into the future. 20% of respondents expect to favour “a balanced mix of commercial, proprietary and open source tools and technologies in building generative AI solutions”; 32% will prefer “commercial tools and technologies (developed by third-party companies) with some open source and/or in-house elements; and 23% will back “open source tools and technologies with some reliance on commercial and/or [in-house] components.”
But also, just under a quarter of respondents expect to be using mostly internally-developed tools and technologies, up from 4% today. That’s a big shift.
In addition to seeking to customise models, competitive differentiation will also be realised by using more enterprise-specific data and knowledge to train and feed the models.
Organisations that use their own data to drive competitive advantage from GenAI use must consider two dimensions as they architect their approach: what data is being used, and the proposed approach to incorporating the data.
Just shy of half of all organisations are looking to use only or primarily internal data to feed the model, while about 40% plan to use a “balanced mix” of internal and external data.
One of the main techniques that will come into play here is Retrieval-Augmented Generation or RAG. This technique improves the quality of text generated by LLMs by incorporating information from corporate knowledge sources. The text is captured before being submitted with the original prompt to LLMs that will originate the final output, where information from inside corporations will still be kept safe and not exposed outside of the context of the task being performed.
The Hitachi-ESG report found that 86% of organisations will lean on RAG to some extent to incorporate enterprise data into their GenAI efforts, in the hopes of locking into a real competitive advantage.
A data platform, underpinned by a certain calibre of infrastructure, will be required to support this strategic shift in direction, with hybrid cloud emerging as the preferred option from an infrastructure perspective.
A combination of on-premises and public cloud, hybrid cloud is preferred by 78% of organisations for building and deploying GenAI solutions. This preference extends to data pipelines used for moving and managing data. This also suggests the need for hybrid cloud data platforms to manage a vast variety of data types including structured and unstructured, and to deliver on the performance and security needs amplified by the anticipated role of RAG.
Good decision-making on the support IT infrastructure can also have a key difference on the performance, reliability and availability of GenAI models, and the energy consumption used by the associated compute and storage. These factors become even more pronounced when GenAI is at the core of delivering a competitive advantage delivery, highlighting the need for a careful evaluation of infrastructure to advance with GenAI in 2025.