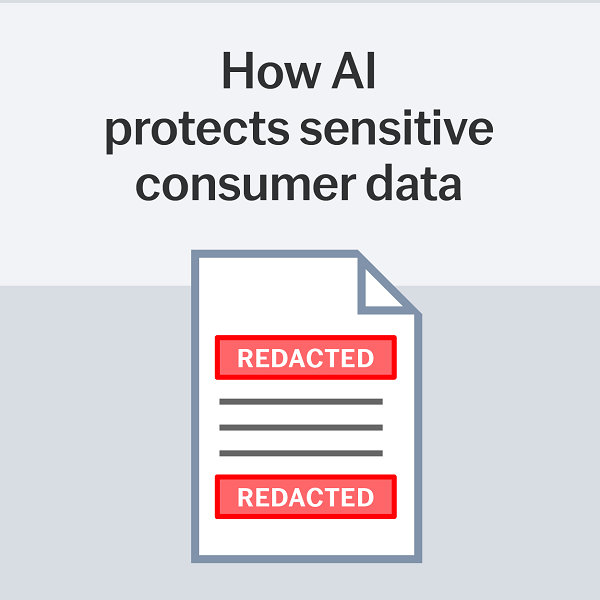
AI helps protect customers’ Personally Identifiable Information (PII) from leaks
By Maxime Vermeir, Senior Director of AI Strategy at ABBYY
Businesses across every industry store sensitive data about customers, from names and addresses to credit card and tax file numbers. This data is categorised as Personally Identifiable Information, or PII, and is typically subject to stringent regulation due to risks of identity theft, privacy violations, and other adverse impacts of this information falling into the wrong hands.
In Australia, PII is stored in vast quantities across millions of documents and is required to be stored for at least seven and up to 45 years according to AUSTRAC Record Keeping guidance, creating potential for catastrophic consequences if compromised.
To encourage Australian businesses to more responsibly safeguard this consumer data, recent legislation has imposed massive financial penalties for data breaches that can reach up to $50 million. As large organisations continue to experience breaches of considerable magnitude, this risk should be top-of-mind for business leaders.
Properly scrubbing PII from stored documents, however, is no easy task. With poor scan quality, chicken-scratch handwriting, varying document formats and other frustrating obstacles, performing thorough PII redaction can pose a major organisational challenge.
With manual PII redaction a near logistical impossibility and data breaches a far too expensive liability to ignore, businesses in Australia are caught between a rock and a hard place – but recent advancements in artificial intelligence might be their ticket out.
AI-enabled scanning, identification, and redaction
Artificial Intelligence (AI) can be used to autonomously identify specified information in scanned and electronic documents alike through several methods of detection. While each method relies on AI-enabled optical character recognition (OCR) to identify and process the text, each varies in complexity and flexibility.
The first is document-specific redaction based on field coordinates. This is the simplest and most template-based method, identifying information in a pre-determined location on a document. For example, if a business has a proprietary invoice format where “Card Number” is in the same place every time, AI can be used to extract the value from that specific location.
This is highly effective for proprietary documents where the contents are predictable and consistent, but automatically redacting PII at a more general scale will require a more flexible approach.
Keyword-based redaction, by contrast, is a document-agnostic approach that relies on specific keywords rather than coordinates. By referring to PII-specific fields with labels like “Card Number” or “CVV” and using OCR to scan the nearby sequence of digits for certain criteria such as “16 numeric characters grouped by 4,” information can be identified and redacted with more flexibility to varying document formats.
Machine learning for field identification and handwriting recognition uses advanced neural networks that identify image fragments with handwriting, which is useful for signatures and other fields that are typically written by hand. These fragments are then passed through the OCR engine to recognise the content within, which will be either redacted or ignored depending on their closeness to redaction criteria. The machine learning component of this technique allows for a feedback loop enabling continuous training and improvement by operators to increase efficiency over time.
Combining all three of these methods in an advanced OCR platform allows businesses to eliminate the extensive manual efforts for data verification by up to 98%, thanks to recent leaps in quality of neural networks and handwriting recognition.
Affected industries, use cases, and incoming legislation
Personally Identifiable Information (PII) is a broad descriptor that encompasses many types of consumer information, making it applicable to any business that stores their customers’ data. Whether it’s a gym membership registration form or a loan application, documents of any magnitude can contain data that could spell disaster if leaked.
Here are just a few examples:
- Banks and lenders – statements, pay slips, rental agreements
- Insurance providers – applications, pay slips
- Government services – personal files, health records, criminal records, employment contracts
- Healthcare organisations – health records, transaction records
- Educational institutions – student records
- Gyms and fitness clubs – direct debit applications
- Real estate organisations – rental agreements, pay slips
PII in government-held documents mandates yet another layer of responsibility due to the Freedom of Information Act of 1982: if someone requests access to a government-held document that contains information about them, PII of other parties must be redacted. Meaning, if you request a court document from a case you were a plaintiff in, PII of jury members must be excluded.
Australian organisations haven’t seen the end of privacy regulation, with Privacy Act reforms expected to be introduced this month. These changes could introduce a tier system for penalties based on the severity of data breaches, as well as higher transparency of PII use in “substantially automated decisions with legal or other significant effects.”
With the wide-reaching implications of storing PII coupled with a constantly evolving legislative landscape, exploring AI-enabled methods to more efficiently and effectively automate PII redaction should be a top priority for business leaders in Australia. It won’t just protect companies from millions of dollars in potential losses – it fulfills their social responsibility to protect consumer data.