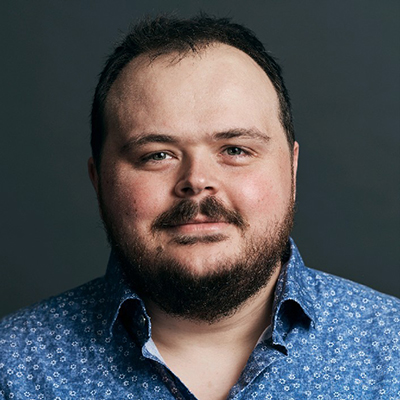
AI, Lakehouse Architecture and Customer Data Management
By Caleb Benningfield (pictured), Head of Lakehouse Strategy at Amperity
‘Lakehouse architecture’ is the perfect blend of a data lake and a data warehouse, offering a unified platform that supports a wide range of data processing and analytics needs. It’s versatile enough to handle both structured and unstructured data.
Eliminating Data Silos in the Age of AI
Traditional data setups often create data silos due to the use of multiple big data environments. These silos can lead to inefficiencies and complicate data management due to the separate storage and the work to copy data between them. For instance, a brand might use different platforms for various purposes—one for database workloads, another for Artificial Intelligence (AI) and machine learning (ML), a separate one for marketing data, and another for financial data. This results in significant data fragmentation. Managing and consolidating these environments typically requires complex migration processes, which can drain IT resources and compromise the effectiveness of each tool.
Lakehouse architecture addresses these issues by enabling live data sharing without the need for complex ETL processes. Data stored in open formats like Delta Tables, Iceberg Tables, and Parquet files can be shared across platforms without copying, allowing for seamless cross-platform workflows and reducing time lost in data transfers. By using open formats and standardised protocols, lakehouse architecture allows for consistent data sharing across different platforms and tools, helping to maintain high data quality and reducing discrepancies that can arise from using multiple, isolated data systems.
Consider a retail company using separate systems for inventory management, CRM, and marketing analytics. Each system holds valuable data, but accessing and integrating this data across platforms can be a logistical nightmare. With a lakehouse architecture, data from all these systems can be stored in a unified, accessible format, allowing seamless data integration and real-time analytics.
Maximising Flexibility and Building the Best Tech Stacks
Another key advantage of lakehouse architecture is its flexibility in building ideal tech stacks. Brands can pick the best tools for specific tasks without worrying about data replication or quality issues. IT teams can select the optimal tools for their needs, using different platforms’ strengths while maintaining data integrity and quality through open formats and protocols. This means all applications consuming the data simultaneously benefit from the improved data quality, enhancing overall performance and efficiency.
Open formats and protocols in lakehouse environments ensure persistent data quality across the tech stack. Data can be shared through a lakehouse catalog and accessed by any tool using the same architecture. This eliminates the need to copy data from one tool to another, maintaining data integrity and reducing the cost and effort associated with data processing and storage.
For example, a marketing team might prefer a specific analytics platform for customer insights, while the finance team relies on another tool for financial reporting. With a lakehouse architecture, both teams can access the same underlying data without creating cumbersome data migrations or duplicates, ensuring that each team gets the accurate, real-time data they need to perform their tasks effectively. This unified approach streamlines operations and sets the stage for significantly improving data quality and governance. It’s like a lake vacation where some are fishing, some are jet-skiing, and some are just hanging out on the dock. Everyone experiences their ideal activities, creating a harmonious and quality time for all.
Real-World Example of Brands Using Lakehouse Architecture
Now, let’s look at a real-life business case. Virgin Atlantic, a leading transatlantic airline network, with connections to over 200 cities around the world, is one example of a brand using lakehouse architecture to transform its customer data approach. The innovative airline combines Databricks with a Lakehouse CDP to unify and enrich its vast customer data. This powerful combination democratises data access, allowing non-technical users to make data-driven decisions quickly and efficiently, maximising the value of customer data for delivering exceptional travel experiences. Virgin Atlantic has improved its data management efficiency and enhanced customer experiences by leveraging real-time insights and personalised interactions made possible through a lakehouse environment.
As more brands adopt this architecture, the potential to enhance data-driven decision-making and improve customer experiences will grow. Brands looking to stay ahead in the data-driven landscape should consider the benefits of lakehouse and open sharing to enhance customer data strategies.
Transforming Data Management
Lakehouse architecture is revolutionising customer data management by eliminating data silos, maximising flexibility, and improving data quality and governance. Major industry players are already embracing this trend. Snowflake’s launch of Iceberg tables, along with similar initiatives by Azure, Google, Salesforce, and Adobe, underscore the transformative potential of lakehouse architecture.
It sets a new standard for how brands handle customer data, enabling advanced AI applications, personalisation, and overall data management efficiency. As the adoption of this architecture continues to grow, the ability to access and integrate data seamlessly across various platforms will become a critical advantage for brands looking to dive ahead in the competitive market.
You don’t have to believe in magic to agree that this transformation and its potential for enhanced efficiency, better decision-making, and improved customer experiences is pretty magical.