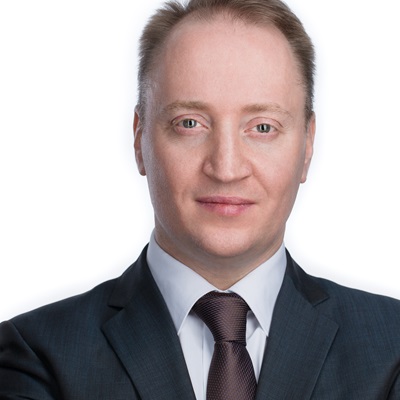
Australia and New Zealand’s industrial sector has a complex relationship with generative AI
By Alexey Lebedev (pictured), Vice President – Pacific at AVEVA.
The ‘quick wins’ found in more corporate and head office functions can’t be easily replicated or translated to the production and processing parts of operations but human insight can certainly help.
Australian and New Zealand industry, from minerals exploration to mining and manufacturing, is showing a keen interest in generative AI. There are even some basic chatbot-style use cases in production, and operators are drawing up their own plans for experimentation and potential use.
But industry generally is likely to have a very different relationship with generative AI than more business-like corporate units or functions.
In the more administrative parts of organisations, even those in heavy industry, generative AI seems able to meet many business needs. The automation in these business units is more straightforward, and so it’s where a lot of the ‘quick wins’ for generative AI exist. According to consulting firm McKinsey, three-quarters of generative AI’s value will be realised in customer operations, marketing and sales, software engineering, and research and development.
It’s a different story when it comes to operations, where creating enduring value will require companies to look beyond quick wins and low-hanging fruit.
On its own, generative AI cannot spark the kind of revolution stakeholders have been led to believe is around the corner. But industry can still ‘produce the goods’ when it comes to finding generative AI-powered ways to unlock value.
To effect lasting change, industry needs to be able to blend the large language model (LLM) “brains” that power generative AI tools – think those made by OpenAI, Google, Meta and others – with real-time up-to-date industrial data and additional, customised, fit-for-purpose AI (such as neural networks and monitoring agents).
It’s only by combining these three technologies with human insight that operators can unlock their true transformative power and create a new realm of value specific to the industrial world.
Industrial use cases
AI systems that are purpose-built for highly specific industrial applications are delivering results today.
Industrial AI is already rapidly transforming sectors like mining, manufacturing, energy, and transportation.
In process manufacturing plants, AI monitors production in real-time, preemptively identifying potential issues and providing guided remediation steps to optimise overall equipment efficiency.
AI data analysis also improves supply-chain management by optimising inventory, preventing shortages, and identifying inefficiencies that affect profitability. Any industry that needs to make fast decisions based on massive amounts of data benefits the most.
For example, in oil and gas, a huge variety of changing information—delivery delays, equipment failure, or even the weather—affects refining schedules. Industrial AI can incorporate all that information as it changes to quickly readjust the refining schedule and ensure it remains safe and effective. Other industries, like power generation, food and beverage, or consumer packaged goods (CPG), use AI to monitor equipment performance and identify equipment problems in advance of any failure.
Organisations are also using industrial AI to enhance net zero ambitions, reduce greenhouse gas emissions and energy consumption, while increasing circularity and efficiency by optimising planning and scheduling.
This is an area where incorporating generative AI technology could enhance the overall effort and impact. The various AIs could be tasked at a high level with minimising industrial greenhouse gas emissions using legal, ethical, and safe methods. More specifically, generative AI could be useful in monitoring the shifting sands of legal, ethical and safety rules and regulations, and alerting to any deviances or changes that might affect the application of industrial AI to operations.
The path forward
But where is the cross over between industrial companies and corporate units using generative AI and LLMs? It is in the crucial area of mitigating challenges around the technology. Lessons learned on one side are directly transferable to experiments on the other.
Hallucinations – where LLMs provide convincing but incorrect responses – are a major issue for all experimenters. Public-facing GPT models like ChatGPT are improving, and the right prompt – search string or phrase – can elicit much more useful responses. But in saying that, it’s unlikely that industrial companies will use these, and will instead want a more controlled and locked-down version of generative AI.
We’re seeing more of these controlled versions emerge; Microsoft’s new Copilot, for example, is architected to protect tenant, group, and individual data, while taking advantage of existing Microsoft security, compliance, and privacy solutions.
An increasing number of software vendors are also integrating controlled generative AI and LLMs into their offerings. In this way, organisations can get quick, out-of-the-box generative AI solutions that are fit for purpose with the ability to scale as needed. The most useful solutions combine LLMs with up-to-date data from additional AI technologies such as neural networks and monitoring agents to ensure data dependencies remain up to date and contextual for maximum, real-world value.
The ability to run production data through generative AI isn’t just important to producing actionable results – it can also reduce potential hallucinations as well. By combining GPT models with up-to-date industrial data (through additional AI techniques), incorrect answers are greatly reduced, with significantly improved results in the industrial context.
Security must also be addressed, and for critical operations, a customer’s data must never be blended with any other customer data or with the LLM.
Still, the operator will need to rely on the LLM creator (or the tool containing the LLM) to keep working on their end to improve the transparency and accountability of the model, making decisions explainable to allay fears about the validity of the course of action it suggests. Practically speaking, human expertise will play a critical role in the initial stages before AI can do any heavy lifting.
The exciting path ahead is filled with possibility – but it is only in addressing the challenges around generative AI that we will unlock its transformative power.