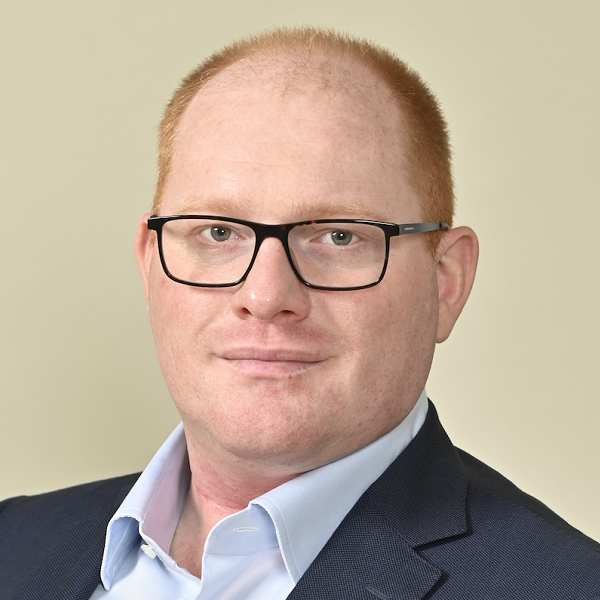
Australian backing of quantum computing is crucial for AI’s future
By Justin Hurst (pictured), Chief Technology Officer APAC at Extreme Networks
Australia has long been recognised as a leader in the quantum space, and governments nationally have demonstrated they want to maximise the potential of the technology as it evolves.
A key emerging area of potential is how the power of quantum computing might be harnessed to advance Artificial Intelligence (AI) beyond what it’s capable of delivering today.
Artificial intelligence, particularly generative AI, is driving the creation of new workflows across enterprises as well as governments and other public organisations. According to one recent survey, AI is 2025’s leading technology trend in Australia. The same survey found “35 per cent of Australian tech leaders think that using AI to drive operational efficiencies is the greatest opportunity for Australian business, an increase of 15 percentage points” year-on-year.
There is undoubtedly a lot of value left for Australian organisations to realise from their AI investments. But keen adopters of AI, of which there are many, are likely to have already come up against some limitations in their current work, including model inefficiency and hardware limitations.
Model inefficiency has been in focus through the first part of 2025, in part due to the emergence of lower cost, energy efficient AI models.
So far, the response to model inefficiency has been to throw more compute capacity at the problem, in part by building more data centres. Data centres catering to high-intensity AI workloads have sprung up domestically and in the region, but need to be co-located near significant power infrastructure. The environmental and financial impact of continually throwing more compute resources at AI raises questions about whether or not this is the right direction to go in, or if better solutions exist.
The relationship between AI models and the compute capacity required to run them is already showing signs of being out of sync. The exponential growth of computational power and the amount of data being generated has fuelled rapid advancement of AI to date, enabling it to tackle increasingly complex tasks, but AI’s progress might just be hitting a wall. Already, mega large language models are not providing the increased performance improvement that Moore’s law gave as a guide for computing up to now.
It’s becoming clear that the next leap in artificial intelligence requires a rethink of the very nature of computing itself.
The hardware evolution
AI has undergone significant transformations since its inception, evolving from basic machine learning models to sophisticated generative AI systems. At its core, AI is designed to run on traditional computer hardware, initially using Central Processing Units (CPUs) and memory to perform operations in a single-threaded manner. These early models stored all parameters in memory, which limited their ability to handle complex computations and large datasets.
As the demand for more advanced AI capabilities grew, the limitations of CPUs became apparent. This led to the adoption of Graphics Processing Units (GPUs), which offered parallel processing capabilities. GPUs could handle multiple operations simultaneously, making them ideal for training and running neural networks. This shift was particularly crucial for developing deep learning models, which require extensive computational power to process vast amounts of data and perform numerous calculations concurrently.
Looking to the future, the potential of quantum computing looms on the horizon. Australia is hoping to have a utility-scale quantum computer on domestic soil as early as the end of 2027.
Quantum computers have shown the benefit of instantaneous parallel processing, which is precisely the issue with large AI models. As the technology progresses, it will be exponentially faster than traditional computing, unlocking entirely new opportunities for AI development.
As quantum hardware improves, the ability to scale up and handle larger datasets and more complex models will surpass the limitations of classical GPUs. Quantum computers can process multiple possibilities simultaneously, offering exponential speed-ups. They also have the potential to be more energy-efficient than classical supercomputers, which is crucial for sustainable AI development.
The case for convergence
The real value of quantum computing will come from its convergence with AI.
The convergence of AI and quantum computing promises to revolutionise technology advancement. Done right, it has the potential to really transform industries, solve complex problems, and drive unprecedented advancements, just as generative AI has suddenly led to a previously unimaginable new age of innovation.
As quantum technology advances, the costs associated with quantum computing are expected to decrease, potentially reaching levels comparable to high-memory GPUs used in AI today.
This convergence will make quantum computing more accessible and practical for a broader range of applications. It will enable AI models to process and learn from data at unprecedented speeds, bringing us closer to the realisation of general AI, where machines can understand, learn, and perform tasks with human-like intelligence.
Focusing on the development of secure and private edge services can significantly support the advancement of local general AI systems, leveraging the unique capabilities of quantum computing. By deploying quantum computing at the edge, organisations can harness the inherent randomness and immense processing power needed for general AI to process local inputs in real time.