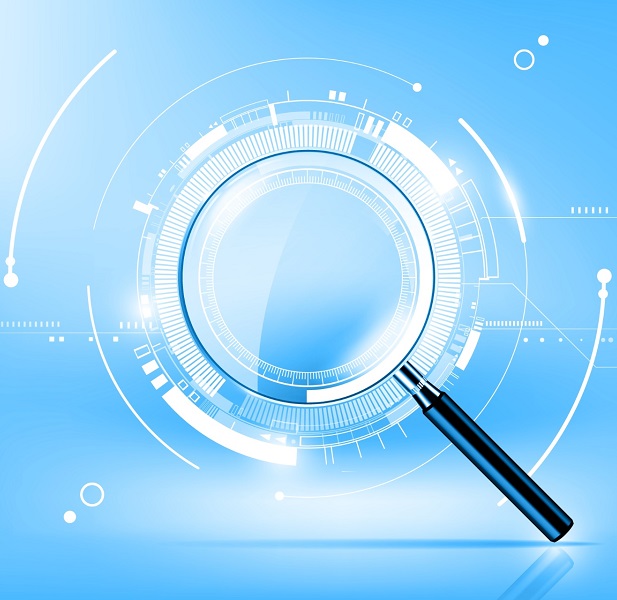
Digital detectives: How AI is overcoming financial crime
Jonathan Tanner, Senior Director of Financial Services & Insurance APAC, Pegasystems
As financial crimes and fraud escalate nationally, the banking sector finds itself in a race not just against criminals, but against the limitations of their own legacy systems.
As a former banker deeply involved in digital transformations, I have witnessed firsthand the evolving landscape of financial crime and fraud. As it stands, traditional financial crime and fraud detection systems are being pushed to their limits. While detection and monitoring technologies have advanced, banks are struggling with the sheer volume of alerts and the considerable operational effort required to manage them effectively. The siloed nature of risk management teams across departments, often employing disparate monitoring and case management tools, only adds to the overall challenge.
Today, these institutions find themselves at a crossroads where the traditional models of managing fraud and financial crimes, involving separate systems that operate independently within financial institutions, is being challenged. This separation was once logical, but as the lines between different types of financial crimes blur, a more integrated approach has become necessary.
So how can banks fight back against the financial crime wave, breaking down the silos and reducing the amount of manual effort and judgement required?
Breaking down silos with artificial intelligence (AI) and machine learning (ML)
From my experience, the challenge begins with the traditional structure of risk management within banks, where teams operate in isolation, often using varied tools that hinder effective communication and data sharing. Tools and strategies such as unified AI-driven platforms that not only enhance detection but also streamline decision-making processes, helps to ensure that insights and data flow seamlessly across all organisational levels.
The adoption of Artificial Intelligence (AI) and Machine Learning (ML) in financial crime detection has led to substantial improvements in several areas. Firstly, these technologies have significantly reduced the rate of false positives. AI systems learn from vast amounts of data, improving their accuracy over time and thereby enhancing the quality of detections and alerts.
Furthermore, AI and ML enable the automation of numerous processes within the detection and investigation phases, eliminating the need for manual intervention and allowing human experts to focus on the more complex aspects of financial crime management. This shift not only boosts efficiency but also helps in reducing operational costs and expediting response times to potential threats.
The benefits of a unified approach
Many of the detection systems in place within financial institutions are purpose-built for anti-money laundering and financial crimes. As schemes become more sophisticated and new digital channels and rails emerge, financial institutions are struggling to aggregate these risks at an enterprise-scale.
Detection systems have various scoring methods to predict the potential risk and priority of alerts. This is very useful, however, multiple scores from multiple detection systems present a challenge. A unified workflow and case management system supports industry best practice, which is to aggregate scores from various detection systems then rescore to factor in risks identified by the multiple systems.
As detection systems incorporate more sophisticated AI and ML analysis into their output, the number of false positives will over time decrease. However, as we continue to see the level of sophistication of the criminals involved in the prosecution of financial crimes increase, the number of investigations required is only going to increase. A unified workflow and case management system helps banks automate and streamline investigative tasks and processes, such as quickly analysing related internal, third-party data, associated parties and alerts. This allows the bank to look at the investigation from a holistic perspective not just based on an individual event, aiding the bank in understanding the risk much more quickly, as well as keeping regulators better apprised of any developing patterns of risk.
The road ahead
As financial institutions continue to grapple with the complexities of modern financial crimes, AI and ML stand out as crucial tools that can tip the scales in their favour. The future of financial crime management lies in leveraging these technologies, complementing the skills of the operational teams, to develop an integrated, intelligent framework that not only detects and prevents crimes more effectively but also enhances the overall efficiency of financial institutions.
As we move forward, adopting AI and automation is not just about keeping up with criminals or regulatory demands; it’s about setting a new standard of operational efficiency and safety in the financial industry.