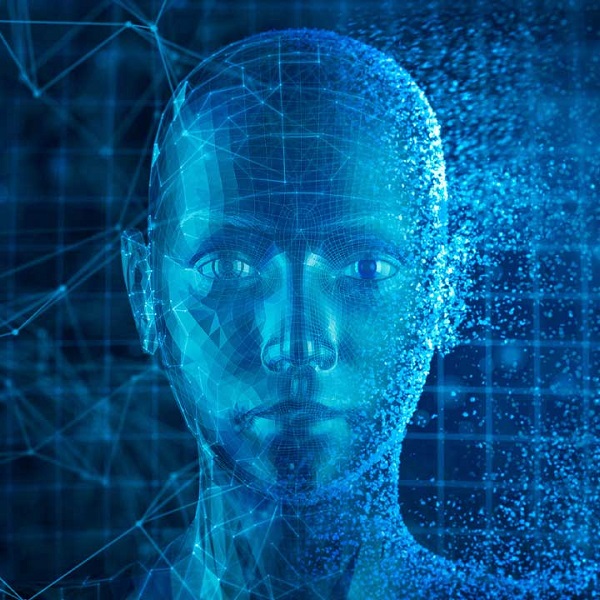
From buzzword to reality – How AI is powering video technology to augment human performance
By Dr. Barry Norton, VP of Research, Milestone Systems
It seems like Artificial Intelligence (AI) dominates the news in ways that few other technologies have managed. And, as awareness of AI has grown, the technology is subject to greater scrutiny as users seek to understand how it is best used. What, if any, threat does its ‘intelligence’ present? How will it interact with human beings? Will it augment people in the workplace, or replace them?
Data-driven video technology – powered by AI – may provide answers to these questions. Driven by AI, video is already serving valuable real-world functions in surveillance applications by taking visual information and structuring it in a way that people can use.
This achieves two things: firstly, it delivers new operational insights by identifying patterns invisible to users. Secondly, in so doing it cements people’s role at the heart of AI, by informing and enhancing their decision-making.
Video pioneers the use of AI
At present, much of the excitement around AI concerns its potential. Yet one industry that made an early leap from analogue to digital by pioneering IP, digitization – and now AI – is already delivering insights and efficiencies to users, using AI: video technology.
This represents a shift from a world in which people are processing raw data – or doing ‘the heavy lifting’ – to one where people are at the centre of the process, creating value out of processed raw data provided to them.
In this new world, people are supported by insights – gained by AI – that give structure to the visual data generated by video cameras. Once used solely for security applications, by structuring visual data video technology it is now as likely to be used to gain operational insights and enhance efficiency as maintaining safety.
Supporting decision-making
In data-driven video technology solutions, technology supports human operators by converting video data into information in the form of structured data that both describes people and objects in a scene, as well as their behaviours and relationships. The human operators then need to focus only on parts of such information obtained from processed video data that are relevant, such as an incident or event.
The vast amount of visual information generated can also be analysed using AI to uncover patterns, trends, and correlations, and these are used to create insights and actionable intelligence that help people make informed decisions. Increasingly, these insights are what the users of video surveillance systems value as outcomes, rather than the images captured on video themselves.
Crucially, at the centre of this technology people serve as the essential ‘human-in-the-loop’, using their skills to verify the analysis provided to them, and make better informed decisions on what actions to take.
Understanding analytics
Basic video analytics include fundamental functions like object detection, recognition and tracking, to name a few, and these are relied on in security applications around the world. For example, in spatial domains, object detection has been used for counting people, protecting perimeters and identifying when objects cross defined lines. In the so-called ‘temporal’ domain, object tracking is used to extract information relating to the trajectory of objects, to assess the direction of moving cars in traffic, for example.
Second-level analytics addresses the interpretation of those objects and their behaviours across frames, making action recognition, interaction detection and anomaly detection all possible. Importantly, these analytics do not have to be focused on an object alone: so-called ‘reconstruction-based’ anomaly detection can in fact work with an entire frame of video. This makes it possible for use in life-saving applications, like detecting when people fall.
Predictive analytics should be the next exciting development in video analytics. Building on top of the insights collected from a given history of objects’ behaviours, likely interactions between objects can be predicted, and it will become possible for security teams to predict eventualities and therefore manage incidents – before they actually occur.
Challenges for the future
Future developments in data-driven video analytics are intrinsically linked to the development of AI. In turn, future advances in AI are subjects to two elements currently in short supply: chips, and data.
The development of AI is dependent on the availability of chips that allow the intensive computing required, yet demand for these chips is such that scarcity is becoming an increasing issue for the AI developers. TSMC, the world’s largest contract chipmaker, predicts that demand for AI processors that perform training and inference functions will grow at around 50% CAGR over the next five years, and the company has described demand for chips as “insatiable.”
Another shortage relates to data itself. Advanced video analytics relies on large, annotated datasets on which to be trained, with corresponding rights for ethical use. Ready to use datasets are in short supply, while the cost of creating and labelling new datasets can be considerable.
A solution to this lies in the use of ‘synthetic’ data. Artificially generated or augmented, synthetic data provides a means of increasing the amount of data available on which to train an AI model. This in turn reduces the need for manual annotation and extensive data collection and delivers training data that more fully represents the diversity of human experience. Importantly, synthetic data can preserve real-world characteristics and safeguards privacy, while also avoiding consent-related issues.
Lifelong learning
The evolution of data-driven video technology, and the development of AI on which it is now reliant, is not only based on the availability of sufficient computational power and data sets. Development and refinement of AI in the future must bring increased reliability and accuracy, and this will, in part, come through fine-tuning models based on their application in the real world. This presents a challenge to developers in how they bridge the gap between testing and live operation, to ensure greater degrees of accuracy in the analyses AI makes.
This can be achieved through the application of ‘ModelOps’, the name given to a collection of tools, technologies and best practices used to deploy, monitor and also manage AI models – including machine learning models, for which the term ‘MLOps’ is employed.’
ModelOps allows developers to uncover suboptimal outcomes in their machine learning models and take appropriate action as required, such as training the model further, or replacing the data it relies on. Using ModelOps, developers can continuously monitor an operational environment and evaluate the performance of an AI model over time. This helps identify and assess any system deterioration, sometimes caused by the changes in data, compared to the training data, as a result of ‘concept drift’ – a corrupting shift in the relationship between the input and output data in an AI model.
Of course, the continued development of AI on which data-driven video technology depends very much relies on the wider acceptance of AI by consumers, businesses and governments. For video surveillance, which has its roots in the use of CCTV cameras for security applications, that trust has already been established. Now that AI underpins innovative applications for data-driven video, however, it’s important that the technology is used in responsible ways.
This requires organisations working with AI to adhere to regulations and, where these are still in development, adhering to guidelines such as the G7 AI Code of Conduct, which Milestone Systems adopted in early 2024. This aims to promote safe, secure, and trustworthy AI worldwide and was agreed by G7 leaders at the end of October 2023 alongside a set of Guiding Principles to follow when developing new AI systems.
Untapped potential
The success of data-driven video technology today owes much to critical stages in the development of AI, including the work of the ‘Godfathers of AI’, the 2018 Turing Award winners Bengio, Hinton and LeCun, whose pioneering work in deep learning drove the development of computer vision, as well as Fei-Fei Li’s development of ImageNet.
Andrew Ng and others found a way to train neural networks at scale using graphic processing units (GPUs), while Google’s introduction of ‘Transformers’ in 2017 advanced work in the field of natural language processing (NLP) and then in computer vision (CV).
Work by these individuals – and many others – has supported the development of data-driven video technology over recent years and has allowed users to gain deeper insights into how their organisations operate, allowing them to make better decisions and gain greater efficiencies.
Now, as AI is developed further, so the evolution of video technology will draw on work in related fields being undertaken today and into the future. As such, the video sector should perhaps be seen as a ‘sandbox’ that delivers real-world applications for AI, providing users of data-driven video technology with value beyond security alone.
The successful application of AI in video technology also provides a signpost for developers, showing how people can and should remain at the centre of the technology. It also serves to remind those that seek to regulate AI that it can be used to support people as they go about their work.
For data-driven video technology and the AI that drives it, it’s most certainly a case of ‘watch this space!’