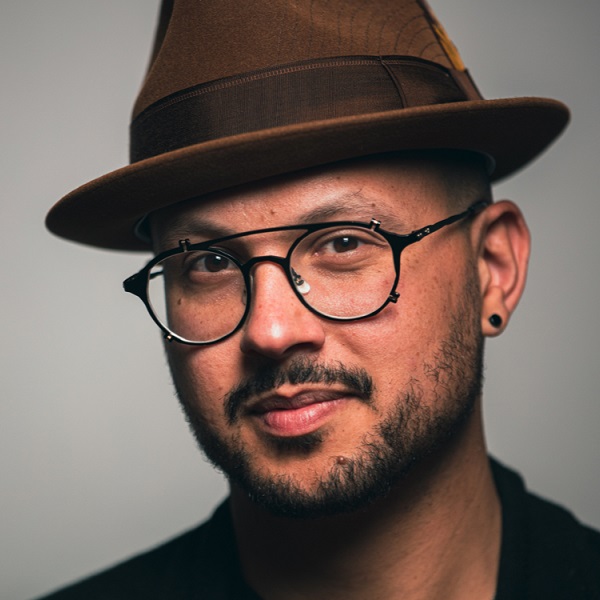
How AI is disrupting how to calculate ‘customer lifetime value’
By Billy Loizou (pictured), Area VP APAC, Amperity
Businesspeople have long realised it’s typically easier to get an existing customer to make a repeat purchase than to win over a new customer and then get them to buy something. That appears to be even more the case in 2024, with widespread agreement that customer acquisition costs are increasing.
And it’s not like it was cheap in the past – this Harvard Business Review article from a decade ago was already warning that “acquiring a new customer is anywhere from five to 25 times more expensive than retaining an existing one”. So how is Artificial Intelligence (AI) disrupting how we calculate Customer Lifetime Value (CLV)? Well before we answer that question, let’s take a look at the past.
How CLV was calculated
It was actually the telemarketers of the 1970s and 1980s who got the Customer Lifetime Value (CLV) ball rolling. After detailed studies of their customers’ purchasing behaviour, telemarketers developed the RFM method – Recency, Frequency and Monetary value. This ‘method’ claimed that data about when a customer last made a purchase, how many purchases they made, and their average purchase amount are indicators of future purchases.
In the mid-1980s, David Schmittlein, presently a Professor of Marketing at the MIT Sloan School of Management, worked out the arithmetic (the Pareto/NBD framework) to turn the lagging indicator of RFM data into forward-looking estimates of what’s now commonly known as Customer Lifetime Value.
Calculations of CLV have become ever more elaborate, mainly due to technological advances. But if you thought sophisticated CRMs and analytics tools had made calculating the lifetime contribution a customer is likely to make to your business’s coffers straightforward, I have some disturbing news for you.
Almost half your CLV attribution could be wrong
According to Amperity Co-Founder and CTO Derek Slager, “What do people get wrong when they’re predicting customer lifetime value? This is an important one; I think there are a couple of really key things that people get wrong. One [is] only focusing on transaction data. What we found in building predictive customer lifetime value algorithms on top of rich, unified customer data is the correlation between the transaction data and all the other data is a really key signal in making good predictions. The other is that building this without a unified view of the customer is even worth the time. It isn’t. We’ve done the math and we found that 46% of customer lifetime value attribution is completely wrong if it’s not done on a unified data foundation.”
As flagged above, accurate estimations of your customers’ CLV helps with everything from segmentation to deciding what marketing campaigns to create for those segments. This raises the question of whether your business’s CLV calculations are as accurate as you believe them to be.
How AI-powered CDPs are changing the CLV game
Your CLV calculations are unlikely to be wildly off the mark, even if you use an ‘old-school’ CRM or CDP. But neither are those calculations likely to be as accurate as they now could be, thanks to rapid advancements in AI. I’ll once again quote Derek Slager, to explain why:
Artificial Intelligence is revolutionising the software solutions that brands use for marketing, particularly around identifying, understanding and connecting with their customers. New advances in AI and machine learning (ML) have unlocked capabilities once thought impossible.
With a CDP, brands can ingest raw customer data across many sources – from online and in-store interactions to loyalty programs, email engagements and financial systems. Once the CDP has captured that data, it uses ML to resolve identities even when records lack unique identifiers across systems.
AI connects essential customer information, including demographics, loyalty, email engagement, and product purchase data, allowing brands with this software to collect richer, cleaner data. This in turn improves ML modelling performance. As a result, brands can use this insight to understand customer lifetime value, enabling them to make strategic decisions related to marketing, customer acquisition and customer retention.
For example, say a cosmetic company is interested in calculating the lifetime value for a particular customer. AI algorithms, such as machine learning models, can analyse vast amounts of data to predict customers’ future behaviours. The software can analyse their past purchases, preferences for online or in-store shopping, frequency of purchases and more, all of which help to more accurately predict their future buying habits. And through these insights, marketers can more effectively reach customers with more relevant ads and product offers, increasing retention and driving sales.
How to collect richer, cleaner data
I can’t speak for all AI-powered CDPs, but I can explain how Amperity works.
To recap, an accurate data foundation is required for accurate CLV calculations. Unfortunately, many brands are still struggling to unify their offline transactions with digital interactions and most are mis-identifying as many as a quarter of their customers.
In the case of Amperity’s AmpAi, AI and ML are used as follows.
AI-powered models predict customer lifetime value (and order frequency, average order size, risk of churn, and product affinity). The predictions made by these AI-powered models are based on a complete picture of the customer that includes full historical data for both online and offline.
That complete picture is assembled by AI and ML matching algorithms that unify online and offline first-party data with the nuance of a human but at massive speed and scale. (A transparent process highlights how and why the AI merged data, facilitating easy audits and ensuring an accurate data foundation.) Also, sophisticated AI matching keeps profiles up to date by accounting for the frequent changes in customer data, such as a new email address, moving to a new home, shifting engagement patterns and preferences, etc.
What CLV calculations should look like in 2024
To recap, the CLV-calculating challenges your organisation is almost certainly facing are maximising data quality and integration, accurately defining the lifetime period, and keeping pace with evolving customer behaviours.
The MarTech presently best placed to help your organisation address these challenges is an AI-powered CDP. I’m not arguing an AI-powered CDP can solve all your organisation’s marketing challenges. However, for the reasons explained above, it’s a powerful tool for unifying and cleansing customer data from multiple touchpoints. And that helps combat the inaccurate or fragmented data that hinders CLV calculations.
Whatever technology it ultimately opts for, I’d argue your organisation’s marketing department, if it wishes to remain competitive, will soon need to be able to do the following:
- Accurately unify the entire transaction history across every channel for each customer. This step is critical to understanding your customers’ transaction history in order to make predictions.
- Determine the time horizon or ‘lifetime’ across which you want to calculate CLV. This will depend on each brand’s typical inter-purchase cycle and goals. For example, mattress brands may calculate CLV over a 10-year period, while grocery stores may calculate CLV over just one year.
- Determine whether you want CLV to be returns-netting and if you want to calculate based on net profit or gross revenue. Most retail brands opt for returns-netting CLV to differentiate customers who frequently buy and return from truly high-value customers.
- Calculate CLV on each customer, then stack-rank them based on their CLV and assign them to different value tiers. Identify the top 1% of customers in terms of CLV, the top 5%, the top 10%, etc. This will allow you to easily compare your highest-value customers to lower-value customers and segment your marketing based on value tier.
- Track CLV on a cohort basis to measure the longitudinal behaviour of customers acquired at different points in time. This can be done at both an overall level and a segment level.
- Ensure your processes, organisational structure and operating model are designed to measure and maximise CLV. It’s critical that your data and technology infrastructure support continuous measurement.
- As your CLV calculation and tracking capabilities mature, consider building predictive CLV models as an additional analytical and segmentation tool. CDPs with sophisticated ML-based techniques can predict each customer’s future CLV, even if they’ve only just made their first purchase!
Personalisation and CLV
The adoption of an AI-powered CDP and the resulting (more accurate) CLV calculations have a range of spin-off benefits. There isn’t space to cover them all here. However, it’s worth noting that better CLV calculations give your organisation a better understanding of the worth of different customer segments, which should facilitate better decisions about marketing budgets, customer retention strategies and customer acquisition costs. On the customer retention side of the equation, wouldn’t you like a clearer view of exactly who your organisation’s biggest-spending customers are so you can double down on your efforts to delight them with personalised interactions and top-notch customer experiences?
CLV is especially important in tough times
I’ll end by returning to a point I made at the beginning of this article – it’s almost always easier to get an existing customer to make another purchase than to win over a new customer. That’s always been the case, but it would seem especially true when consumers are reeling from cost-of-living pressures and scrutinising any outgoings carefully.
Derek Slager recently pointed out, “In a down economy, customer retention becomes life-or-death. For brands and retailers, the ongoing economic crunch and inflation crisis turns up the pressure on every aspect of operations. Businesses are looking for ways to do more with less, cutting costs by any means necessary in order to stay lean during a potential recession. At the same time, consumers have less money to spend with their favourite brands – let alone with new sellers. In this economic environment, organisations will have to shift their focus from customer acquisition to retention. And while many sales and marketing teams have used the data revolution to inform their customer acquisition strategies – targeting potential customers who share attributes with their best existing customers – first-party data will prove even more important for retention.”