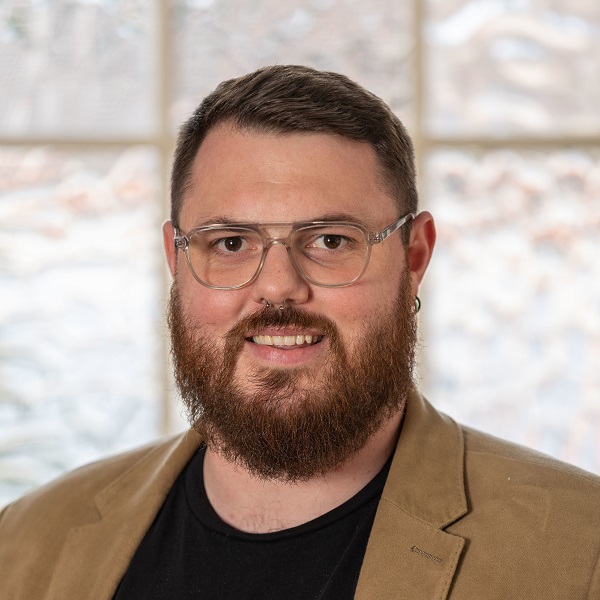
Key security considerations around the use of Generative AI
By Jason Duerden (pictured), Regional Director Australia and New Zealand at SentinelOne
As organisations embrace artificial intelligence (AI) tools at an increasing rate, it will be vital for them to consider the associated security implications.
Capable of streamlining many business processes and supporting staff with everything from copy generation to data analysis, the generative AI tools are being seen as an opportunity to both boost productivity and reduce operational costs.
Interest in AI exploded with the release of the large-language model (LLM) based ChatGPT in late 2022. Since then, companies such as Google and Microsoft have rushed to deploy AI capabilities into a variety of offerings and services.
Opportunities for cybercriminals
While it’s clear that usage of LLM-powered AI tools is still in its infancy, the speed of adoption is showing no sign of slowing. While the focus is, naturally, on the business benefits it can deliver, the implications for IT security also need to be carefully considered.
Just as the technology is being adopted by organisations of all sizes, it has also captured the attention of cybercriminals. They are interested in how AI can be used to improve the effectiveness of attacks to cause disruption and loss.
One early example is the way in which AI tools are being used to craft better-quality phishing email messages. In the past, such messages were often easy to spot as they contained misspellings and poor grammar.
Armed with a tool such as ChatGPT, a cybercriminal can generate large numbers of seemingly authentic messages. This is likely to increase the number that are opened and result in a successful attack.
AI tools can also be used to collect personal data from internet sites. This can then be used to craft spear-phishing attacks that are highly targeted and have proven very effective in the past.
There is also evidence that there are LLM datasets available online designed specifically to aid the activity of cybercriminals. The datasets can be used for pen testing, allowing the criminals to adjust their attack techniques to increase their chances of success.
The advantages for security teams
While this sounds like bad news for IT security teams, AI tools and LLMs can also provide them with some strategic benefits.
For example, LLMs can be used to scour large volumes of logs and other security data to find patterns or particular events that require a change in security strategy. Clues that may have gone unnoticed to a human can be highlighted for further examination.
AI-powered tools can also propose responses based on past events and behaviours. This can lead to the velocity of problem solving by security teams increasing significantly.
At the same time, AI can also make security tools easier to use. Security team members can make requests using plain English rather than needing to understand and remember complex commands and syntax.
The tools also have the potential to assist organisations overcome the ongoing shortage of skilled IT security professionals. By automating some of the more tedious and time-consuming tasks, the tools will free up staff to focus on more value-adding activities. Rather than replacing humans, they will support them to be more productive.
Developing an AI security strategy
For security around AI to be as effective as possible, an organisation’s security team needs to be involved in IT projects from the outset.
They should be given the opportunity to evaluate chosen tools and services to determine whether they are likely to have any impact on existing security measures and what additional elements may need to be added. Starting this process early will avoid the prospect of having to bolt on security afterwards.
An effective AI security strategy also needs to consider what data is being used by the tools, where it is stored, and how it is protected. For example, there will need to be measures in place to prevent staff from inadvertently exposing sensitive data to public LLMs.
Such monitoring will be even more important than it has been previously if an organisation opts to make use of an external LLM tool rather than developing and running one in-house.
The security strategy also has to recognise that the outputs of LLM-powered AI tools are not always accurate. There have been instances where so-called ‘hallucinations’ have resulted in factually incorrect responses to queries.
For this reason, processes need to be put in place to ensure that regular checks are made to ensure suitable quality levels are being maintained. Failure to do this could result in wrong decisions being taken that have a detrimental impact on the organisation.
In many ways, the adoption of AI is likely to follow a similar path to that of the early days of internet adoption. At first businesses were happy to deploy web servers without firewall protection and were unaware of the need for antivirus tools.
Overtime, this changed as senior decision makers became aware of the potential risks their organisations now faced. A similar process is now likely to happen with AI.
By carefully evaluating the security implications of IT usage, organisations can ensure they are well placed to enjoy their benefits while also being resilient against any cybercriminal attacks.