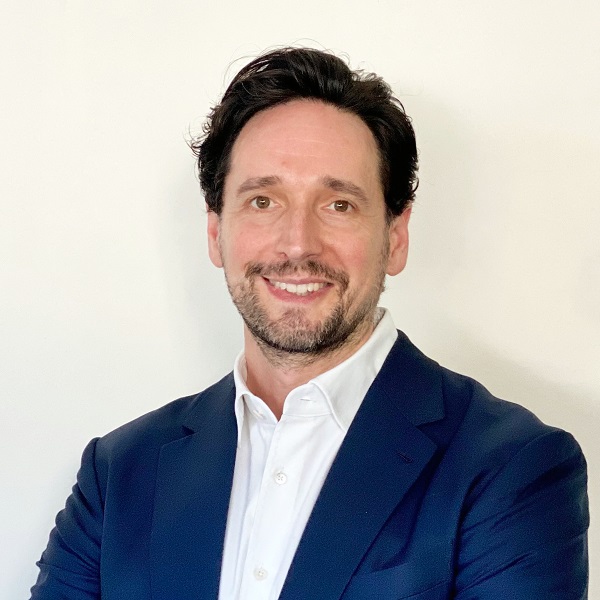
Knowledge graphs are key to delivering responsible and trusted AI
By Peter Philipp (pictured), General Manager – ANZ at Neo4j
Australia’s top two tiers of government recently agreed on a national framework for assurance around their use of Artificial Intelligence (AI). It establishes both “cornerstones” and practices of AI assurance, with the aim “to gain public confidence and trust” by “being exemplars in the safe and responsible use of AI.”
This kind of guidance has been in-demand since the formative stages of Generative AI. As governments and enterprises alike have grappled with AI, the guidance, guardrails and governance around its use has ranged from vague and generic to detailed and proscriptive – and everything in-between.
A key challenge facing organisations today is that the depth and breadth of AI integration into operations, as is now envisioned, leads many to confront two profound ethical dilemmas: around responsible use and trustworthy outputs.
Responsible use is very much a question of ethics. To an extent, this conversation has already played out in many enterprises that have previously gone down the path of big data and personalisation. In large Australian organisations, there is already some clear demarcation around where data use crosses from acceptable to intrusive. Some enterprises, such as banks and telcos, publish proactive guidance on where they sit.
While demonstrating responsibility is one element of trust, trust is a much broader challenge that particularly pertains to AI outputs.
Establishing trust is especially crucial in sectors like healthcare, finance, and autonomous vehicles, where AI decisions can have significant real-world consequences. We know already that humans won’t trust AI unless its results are accurate, transparent, and explainable – to everyone. In addition, users need to know where AI-generated answers come from without requiring a PhD to understand the origins.
While agreement on principles and practices such as Australia’s national framework signal that progress is being made, it – like much international policy for the regulation of artificial intelligence – is still missing an essential element that is critical to its effectiveness and success.
That element is the vehicle that will enable these aspirational guardrails and “cornerstones” of widespread AI adoption to be delivered.
A focus on data systems
For AI systems to benefit humanity, instil trust and meet key regulatory standards it’s crucial to consider the broader technology infrastructure, and most critically, the data systems that underpin the AI systems.
In particular, consideration should be given to the role that graph databases and knowledge graphs can fulfil as a vital data element that enhances AI solutions by providing increased accuracy, transparency, and explainability.
Graph databases provide storage and query capabilities for the analytics, while knowledge graphs enable semantic, relationship-based queries, inferencing and reasoning. Together, they play an essential role of grounding machine intelligence to model human reasoning at a larger scale. They do this by providing contextual understanding, reasoning, and training for machine learning, and making AI outcomes more accurate, transparent and explainable.
Organisations around the world are already using knowledge graphs to train their systems, and unlock new opportunities and more value from their data.
In healthcare, a leading staffing company serving hospitals and health systems leveraged GenAI technology and knowledge graph technology to efficiently match specialised surgeons to diverse job descriptions ultimately improving life-saving patient care.
In energy, a large global conglomerate harnessed the power of GenAI through knowledge graphs to streamline its enterprise knowledge hub by consolidating data from over 250 subdivisions, enabling enhanced predictive analytics, ML workloads, and process automation.
Despite already being widely used by organisations worldwide for mission-critical work, knowledge graph technology is conspicuously missing from conversations with policymakers who are seeking input from industry leaders to build frameworks that safeguard the development and use of AI.
This discrepancy underscores a critical truth about the future of the AI landscape: We need a more systemic, evidence-based approach that brings together the core tenets that will define safe and responsible uses of AI.