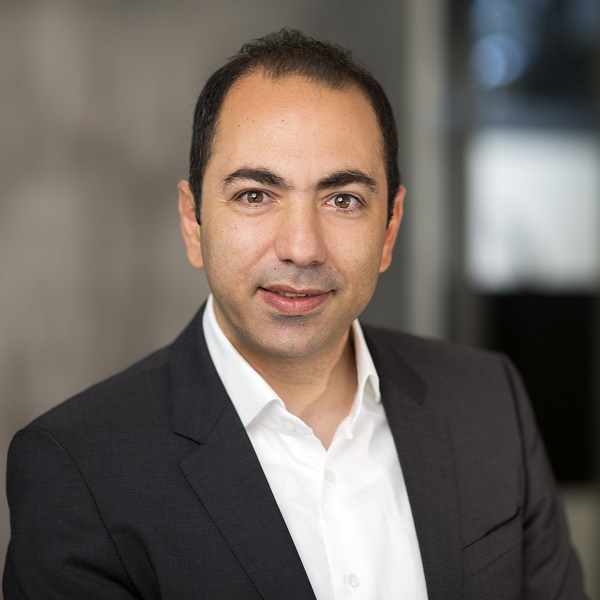
Responsible AI: A cornerstone of trustworthy business decisions
By Rafi Katanasho (pictured), APAC Chief Technology Officer at Dynatrace
Artificial intelligence (AI) is rapidly transforming industries worldwide, with its applications permeating every sector. From automating mundane tasks to optimising complex logistics, AI is revolutionising the way businesses operate.
This trend has been further accelerated by the recent democratisation of powerful tools like ChatGPT, thus making AI capabilities more accessible than ever before. However, as AI systems grow in complexity and penetrate deeper into core decision-making processes, so too does the need for responsible and trustworthy implementation.
A recent Dynatrace report, The state of AI, revealed a significant concern among business leaders. Nearly all (98%) of the 1,300 surveyed technology leaders expressed worries about generative AI’s susceptibility to bias, errors, and misinformation.
These concerns are well-founded. The potential consequences of overlooking key aspects of responsible AI can be severe, leading to financial, operational, and even legal repercussions. Some critical areas that require focus include:
- Opaque algorithms: The inner workings of AI systems, especially those trained on vast and intricate data sets, can be difficult to comprehend. This lack of transparency hinders understanding the basis for AI decisions.
- AI system bias: Both intentional and unintentional biases can creep into AI systems and their data, reflecting the prejudices of the creators or inherent biases within the training data.
- Unauthorised data usage: Organisations must minimise the risk of unauthorised data access by AI systems. This extends beyond a company’s own data to encompass sensitive customer and user information.
Building a foundation
To ensure a responsible AI approach, organisations need to take a holistic view of their IT system-monitoring strategies. This means prioritising accurate, unbiased, and timely data inputs as traditional monitoring methods that rely on siloed data and reactive responses are no longer sufficient.
A unified observability platform serves as the backbone for responsible AI. Such a platform should gather, store, and analyse data comprehensively while preserving context. This contextual data becomes the foundation for training AI algorithms, ensuring they are unbiased, accurate, secure, and up-to-date.
A case study in responsible AI
As a company, Dynatrace itself has a strong commitment to responsible AI. It leverages its expertise in collecting and analysing vast amounts of observability and security data. This data is then transformed into actionable insights, empowering customers to streamline cloud operations and deliver exceptional digital experiences.
At the heart of Dynatrace’s responsible AI approach lies Davis AI, a powerful engine that delivers several key benefits. They include:
- Transparent, explainable AI: Users get full transparency into how Davis AI derives answers and which techniques it has used. Users are in control of each phase of processing to ensure data privacy, eliminate bias, and promote fairness.
- Trusted data: Customers have full control over the data that Davis AI uses. They can choose which data to share that can be used to generate answers. At any time, they can investigate what system data the tool is evaluating.
- Causal AI that’s repeatable: Unlike probabilistic approaches, Davis AI delivers causal, deterministic answers that are repeatable. Causal AI can identify precise cause and effect.
- Data privacy and security: Data privacy principles are embedded into the core of the platform. This gives customers the ability to extend protections beyond the minimum legal requirements when it comes to protecting customer data.
Unleashing the power of responsible AI
Tools such as Davis AI can streamline and optimise IT operations, however these advanced capabilities go beyond simple automation. They can proactively identify anomalies, predict potential issues, and deliver actionable insights that fuel smarter decision-making.
Good tools will have a user-friendly interface, making it accessible to teams across an organisation. Some of the most transformative use cases of responsible AI include:
- Guarding against the unexpected:
Imagine a proactive guardian constantly monitoring your IT infrastructure. Leveraging multidimensional baselining, responsible AI tools can automatically detect deviations from normal performance – fluctuations in application response times, spikes in error rates, or unusual patterns in application traffic and service load. - Pinpointing the Culprit:
Troubleshooting IT issues can be a time-consuming and frustrating task. Responsible AI tools streamline this process by acting as a digital ‘Sherlock Holmes’. When customer-facing issues arise, the tools can utilise a combination of real-time data on application topology, transactions, and even code-level information to pinpoint the problem.
- Predicting the future:
Imagine having a crystal ball for your IT infrastructure. Responsible AI tools predictive capabilities can offer a glimpse into the future. They can anticipate potential issues before they occur, allowing for proactive intervention.
These are just a few examples of how responsible AI can empower organisations to gain a deeper understanding of their IT environments, identify and address issues swiftly, and ultimately deliver exceptional digital experiences.
The future of responsible AI is brimming with possibilities, paving the way for a more efficient, proactive, and intelligent approach to IT management.