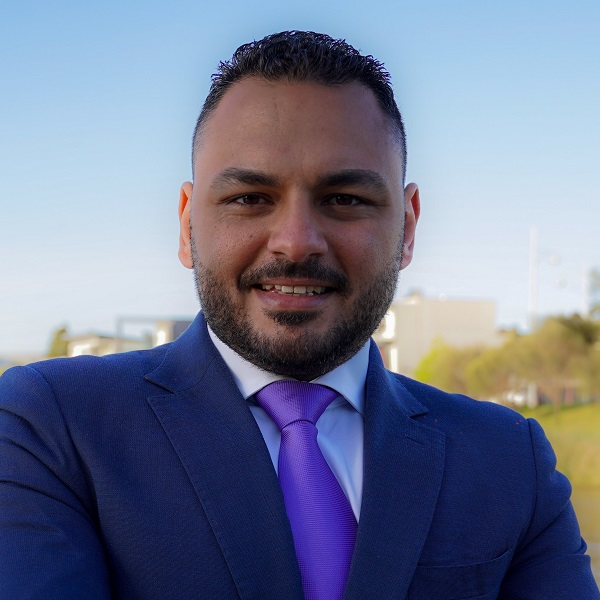
The two things Australian organisations are getting wrong with AI
By Mina Mousa (pictured), Head of Systems Engineering Australia and New Zealand at Extreme Networks
When it comes to Artificial Intelligence (AI), the spectrum of success is broad: stretching from AI being an accelerator for something an organisation already does, to AI creating a new, seemingly magical process. But there is a lot of space between those two points, and the evidence indicates that some Australian organisations are struggling to produce an outcome or result that would sit on that spectrum at all.
The reasons for this have crystallised throughout 2024.
One of the challenges is that some of the conventional wisdom that’s been built up around enterprise AI is either wrong or would be unwise to follow. It’s often said that organisations should begin with ‘low hanging fruit’ or low-risk use cases. Unfortunately, these tasks often lead to inconsequential outcomes that do little to justify the effort and investment expended on AI implementation, let alone put runs on the board that can support the expansion of AI use across the organisation or applied to more critical business functions or processes.
A second related challenge that has emerged is that the focus on creating a too-broad AI strategy may be misplaced. While executives or the Board may put pressure on IT, the existence of an AI strategy does not determine success. Organisations need to consider how they will support the introduction of AI – while building trust and without trying to completely change the company’s culture – if they are to have a better chance of seeing a real ROI on AI investments.
Let’s examine both of these challenges in a little more detail.
Challenge #1: Creating inconsequential AI
One of the most common pieces of advice given to organisations looking to use AI is to start with a low-risk process or use case. There are clear reasons for this advice. One of the things that enterprises are fearful of is AI misuse or mistakes. Compartmentalising the initial use case is a de-risking strategy as much as anything: allowing the technology to be tested while limiting the potential consequences of the test not functioning in an expected way.
The problem with chasing this kind of low-risk use case initially is that something this easy to do is often of limited value. If it was always this easy to fix, that may be the reason no one has done it. Holding this up as the internal example of what AI is capable of is unlikely to win plaudits because whether it’s enhanced or not is inconsequential to day-to-day operations.
The course correction here for organisations is to pick a use case that people care about, because when it is successfully augmented with AI, people will notice, the return-on-investment will be both apparent and material, and it’s more likely to build support for more consequential applications of AI technology.
This is a change from how organisations often treat experimentation with new or emerging technology. It’s counterintuitive and may scare some of the people involved to experiment on a consequential process or function, but this is the nature of working with AI. Picking one or two use cases that matter will allow organisations to get past current perceptions that AI is producing little or no value, and to get onto the spectrum of success where they can either accelerate what they do or start producing some more magical outcomes.
Challenge #2: You need an experience strategy, not an AI strategy
Over the past year, every organisation has likely found itself under some pressure to produce an AI strategy and show how it might be able to benefit from the technology. That pressure comes internally where there’s an appetite to trial AI and see if it has a benefit, and externally where rivals may be talking up their own AI ambitions, driving all those around them to give the technology similar consideration.
But again, AI is different to other emerging technologies that organisations have previously encountered. And while it may seem somewhat counterintuitive, the reality is that the best way to talk about AI is to not talk about AI.
AI is really just a building block – each tool or large language model (LLM) can be thought of as a raw input material. Just as in the construction industry there is no need to have a cement strategy, there is no need for every organisation to have an AI strategy. Instead, the strategy should be aligned to what organisations want the AI to do. This tends to boil down to experience enhancement – of staff, customers and other stakeholders.
So, rather than an AI strategy, it makes sense to have an experience strategy, with AI one of the tools available to accelerate, replace or create new experiences. Accelerate, Replace or Create – ARC – is a useful framework in which to think about an experience strategy. Every organisation has experiences that take too long that they would like to accelerate – for example, customer support. There are also some experiences that would be better if they were replaced entirely, or gaps where there is an opportunity to stand up an entirely new experience offering.
Organisations can then consider whether AI has a role to play to accelerate, replace or create an experience, or whether other technologies would be more useful as experience enablers.
Looking at what experiences you have and want to deliver is a much more manageable problem than trying to strategise the use of a particular technology. AI exists to accomplish something so it makes the most sense to build a strategy on what the organisation wants to accomplish with AI, rather than on AI itself.