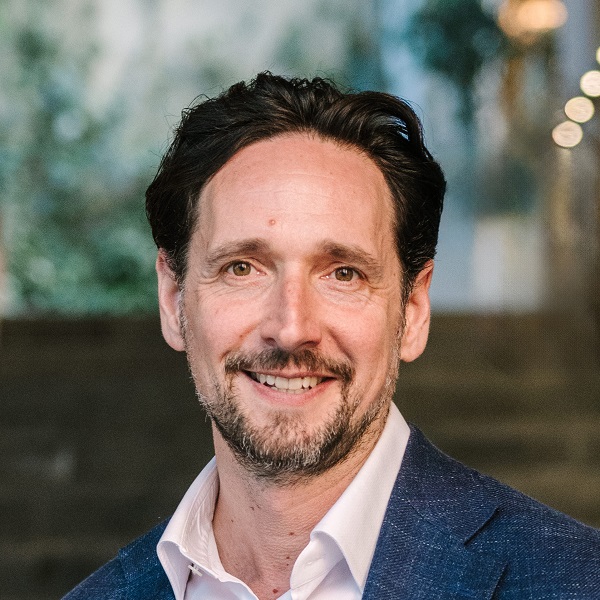
Trends that will underpin the evolution of AI in 2025
By Peter Philipp (pictured), General Manager – ANZ at Neo4j
The use of Artificial Intelligence (AI) tools surged in 2024, and this trend is set to continue throughout the coming 12 months.
Attracted by opportunities for increased productivity and streamlined workflows, businesses are exploring the ways in which the technology can be put to work. Individuals too are using AI to assist with everything from copywriting and image creation to event planning and gift suggestions.
According to research from the University of Queensland almost half (47%) of Australians use AI multiple times each month and 21% say they have incorporated it into their daily routines. Uptake is likely to grow further in 2025.
As the new year unfolds, some of the key AI-related trends to watch include:
1. The rise of ‘agentic AI’:
While generative AI captured widespread attention during 2024, in 2025 so-called agentic AI will rise to prominence as AI agents take over entire workflows and routine tasks. Agent-based AI has ‘chaining’ capability and can therefore divide a query into individual steps and process them sequentially and iteratively. It acts dynamically, plans and changes actions depending on the context, and delegates subtasks to various tools.
During 2024, Anthropic presented AI agents in Claude which operate the computer almost like a human and type, click and surf the Internet for information independently. Microsoft is also launching its own agents, which will perform tasks in sales, customer support and accounting in the future.
While outsourcing routine tasks to agentic AI will be tempting, care will need to be taken to ensure the agents remain controlled. Having operational guidelines in place will be key.
2. Thinking aloud in a ‘black box’:
Although reasoning AI is also not completely new, it is attracting a considerable amount of interest. As with GenAI, LLMs generate answers, but take considerably more time to ‘think aloud’ about the question in a certain way.
The models consider options, draft solutions and discard them before formulating a suggestion. While this takes longer, the quality of the results is also significantly higher.
OpenAI‘s AI model o1 even made it into the top 500 of the US Mathematical Olympiad (AIME) with such logical, mathematical capabilities.
However, reasoning AI does have a problem. The ‘chain of thought’ it uses is hidden in the LLM and cannot be seen from the outside. The so-called ‘loud reasoning’ of the AI therefore actually takes place in silence and significantly undermines its trustworthiness. The increased runtime and costs are also more suitable for individual research tasks than for end users.
3. Usage of small language models (SLMs):
During 2025, organisations will primarily be concerned with using existing AI technologies effectively. Integration is not only a question of compliance and expertise but also a question of cost. As soon as AI is used on a large scale, it is not cheap (just like the cloud).
Also, LLMs trained on publicly available data create little room to differentiate themselves from other users and competitors on the market. Companies are therefore increasingly turning their attention to vertical AIs that are precisely tailored to individual use cases and needs and are continuously refined, optimised, and adapted (post-training).
Instead of large-language models, small-language models (SLMs) are increasingly being chosen, as they can easily compete with the big ones in terms of performance in domain- and industry-specific areas. Their advantage is they can be better controlled and validated (for example via knowledge graphs).
Training with high-quality data is faster, and the models require less than 5% of the energy consumption of LLMs. In addition, good LLMs can also generate high-quality synthetic training data for SLMs, which makes them practically ‘trained’.
4. Data remains at the centre:
While AI vendors such as OpenAI, Anthropic, IBM, and Google are slowly running out of public data, enterprises are primarily concerned with their own data. AI performance depends on organisations’ ability to connect and enrich their own data sets, from Retrieval-Augmented Generation (RAG) and fine-tuning to training their own models. Data quality is, therefore, critical. In most cases, companies have sufficient structured data that already represents the essence of their business.
As important as structured data is, it represents only 10% of the data available. The other 90% is unstructured data such as documents, images, and video files. GenAI, natural language processing, and graph database technology help make this data actionable. Knowledge graphs, for example, represent unstructured data so that LLMs can understand it in context.
5. The evaluation of AI:
Thanks to rapid advances in technology, building new AI applications is now quite simple. Transferring the application into reliable production and validating it, on the other hand, takes a lot of time and effort.
LLMs work probabilistically. That is, they only generate statements with a certain probability. Control and feedback mechanisms are therefore urgently needed to avoid error propagation, check data quality, and meet compliance guidelines.
Unfortunately, conventional approaches often fall short. Instead, AI can be used to control AI. So-called ‘adversarial’ LLMs can, for example, scrutinise the results of another LLM and examine the questions and the answers for any inappropriate or illegal content.
As an example, Anthropic is currently researching so-called interpretable features which are contained in the models themselves and influence GenAI results in a certain direction. If implemented correctly, these tendencies could be controlled and serve as safety mechanisms.
Together, these trends offer insight into what is likely to determine the direction of AI development throughout 2025. With the technology’s evolution showing no sign of slowing, its impact on the world will be significant.