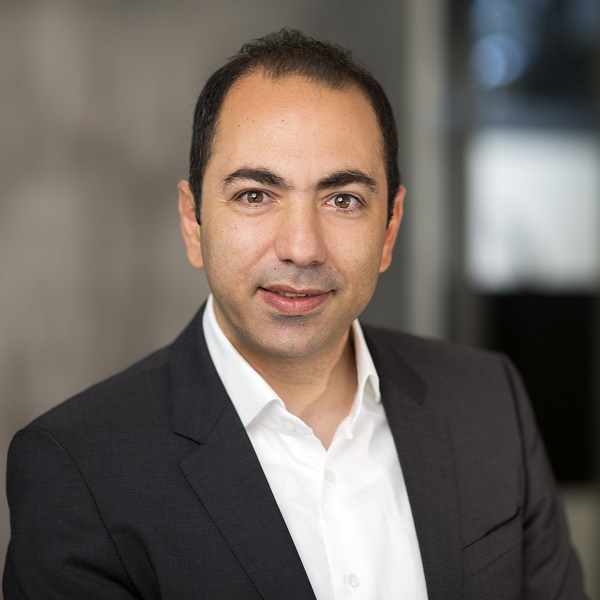
Why taking an ‘explainable’ approach to AI adoption is key
By Rafi Katanasho, APAC Chief Technology Officer at Dynatrace
With the power and usage of artificial intelligence (AI) tools growing exponentially, attention is focusing on exactly how they create their outputs.
The tools can generate anything from written copy and computer code to insights into complex data sets. Automation and analysis features in particular have boosted operational efficiency and performance by tracking and responding to complex and information-dense situations.
AI is already delivering significant business benefits that will continue to increase over coming years. Increasingly, AI will be used to support human decision making and analysis in myriad ways.
However, often it’s not clear exactly how the AI software reaches the conclusions presented and the steps that were followed along the way. As the tools become used in more ways and to undertake important support services, there are calls for better insight into exactly how they are working.
These calls are being answered by an approach dubbed ‘explainable AI’ which aims to make AI output more transparent and understandable. It helps to overcome what has been dubbed the ‘black-box problem’ associated with AI when even domain experts can’t understand how or why a particular model makes its decisions.
The power of explainable AI
In reality, explainable AI can mean a number of different things and so defining the term itself can be challenging. To some, it’s a design methodology and a foundational pillar of the AI model development process.
For others, explainable AI is also the name of a set of features or capabilities expected from an AI-based solution, such as decision trees and dashboard components. The term can also describe a way of using an AI tool that upholds the tenets of AI transparency.
Explainable AI methodologies are still in the early stages of development. Five years from now, there will be a range of new tools and methods for understanding complex AI models, even as these models continue to grow and evolve.
At this point, it is important that AI experts and solution providers continue to strive for effective explainability in AI applications. In this way, they will be able to provide organisations with safe, reliable, and powerful AI tools.
Different approaches to explainable AI will incorporate different components. However, those that offer comprehensive insights will have three key features:
- An ability to interpret:AI predictions, decisions, and other outputs have to be understandable to a human and, at the very least, should be traceable through the model’s decision-making process. The depth of interpretability needed will depend on the particular model being used.
- Insight into communication:The way in which an explainable AI-oriented offering communicates information is also very important. Strong visualisation tools are necessary to fully maximise any explainable AI method’s benefits. These tools can turn data into actionable insights.
- Offers global and local understandability:Global and local explanations have an important distinction. Global explanations are analyses and information that give users insight into how an AI model is acting as a whole while local explanations are insights into an AI model’s individual decisions. Both are important if an organisation needs to understand an odd or incorrect output.
The importance of explainable AI
Overall, explainable AI is an aspect of artificial intelligence that aims to make the technology more transparent and understandable. In an ideal world, a robust AI model can perform complex tasks while users observe the decision process and audit any errors or concerns.
The importance of AI understandability is growing across all industry and business sectors. For example, finance and healthcare applications may need to meet regulatory requirements involving AI tool transparency.
Meanwhile, safety concerns are at the forefront of autonomous vehicle development, and model understandability is crucial to improving and maintaining functionality. Explainable AI is therefore more than a matter of convenience, but also a critical part of business operation and industry standards.
There is also increasing concern about the potential bias and dependability of AI models. Generative AI hallucinations regularly occur, and some AI models have an established history of output bias based on race, gender, and other factors. Explainable AI tools and practices are important for understanding and weeding out biases like these to improve output accuracy and operational efficiency.
Ultimately, explainable AI will enable organisations to gain maximum benefits from what is a rapidly developing technology while also being confident that the outputs being produced are accurate and logical.
Expect to see the topic of explainable AI continue to become more important in the months and years ahead.