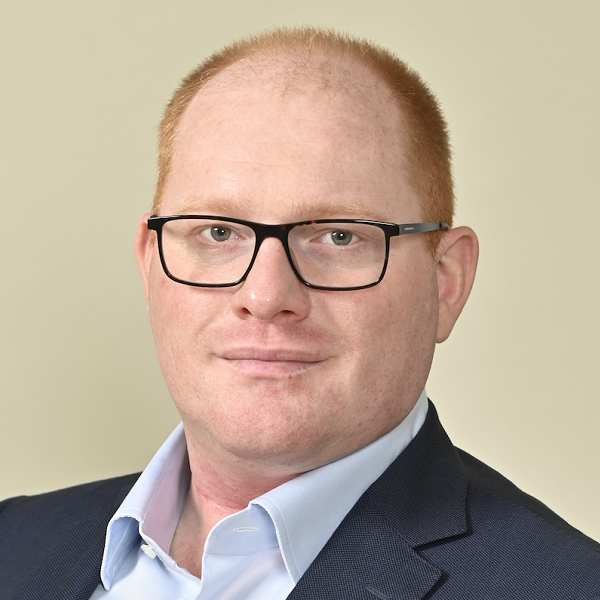
How network engineering is changing in the AI era
By Justin Hurst (pictured), Chief Technology Officer APAC at Extreme Networks
A network outage used to mean engineers scrambling to diagnose the issue–digging through logs, running tests, and manually reconfiguring systems. Today, Artificial Intelligence (AI) can identify anomalies before problems arise and adjust network parameters in real time. The role of network engineers is no longer about firefighting; it’s shifting toward guiding intelligent, self-optimising systems.
AI is no longer just a tool, it’s a critical element of enterprise strategy, transforming IT and reshaping industries worldwide.
At the same time, businesses are increasingly reliant on adaptive systems capable of real-time data processing and advanced computing workloads. To support this shift, networks are evolving from static infrastructures into intelligent ecosystems that dynamically respond to rapidly shifting demands.
At the heart of this transformation is the network engineer, whose role has expanded beyond traditional tasks to encompass strategic decision-making in collaboration with AI.
AI’s role in modern network management
AI is no longer just an automation tool. It has become collaborative ‘co-worker’ for network engineers, working alongside them to analyse vast datasets, identify anomalies, and provide actionable insights.
By offloading routine maintenance and troubleshooting, AI allows engineers to shift from operational firefighting to strategic decision-making. Instead of reacting to network issues, engineers can focus on building adaptive, scalable architectures that support emerging technologies like edge computing and augmented reality (AR).
This transformation requires a new mindset. Engineers must recognise AI as a collaborative partner that enhances decision-making rather than a disruptive force that replaces human expertise. AI-driven networks can adjust traffic flows, prioritise critical workloads, and optimise performance with minimal intervention. However, the real value comes when engineers and AI work together to shape long-term network strategy.
To thrive in this new era, engineers must learn to interact with AI systems, interpret AI-generated insights, and integrate them into infrastructure planning, security policies, and operational workflows. Those who adapt to this new partnership will be at the forefront of AI-driven networking.
Preparing engineers for AI integration
The smooth integration of AI into network engineering practice requires a combination of technical acumen, strategic thinking, and adaptability. Key skills for engineers to work effectively along AI systems include:
- Understanding AI systems: Engineers must familiarise themselves with different types of AI: conversational, interactive, and autonomous.
- Conversational AI streamlines access to data and insights through natural language queries. For instance, engineers can request critical alerts or performance reports via chatbots.
- Interactive AI systems engage in real-time exchanges, providing detailed analyses and visualisations to support decision-making.
- Agentic AI systems, on the other hand, operate within predefined parameters, monitoring, optimising, and even self-healing networks with little to no human input.
- Data literacy: Modern networks and applications generate extensive performance metrics and anomaly reports. Engineers must develop the ability to interpret this data and validate AI-driven insights, ensuring accuracy to build trust.
- Platform proficiency: Familiarity with AI-powered tools offered by vendors is essential. Engineers should understand how to configure these systems and align them with enterprise objectives.
- Adaptability: The rapid evolution of AI technologies demands continuous learning and flexibility. Engineers must stay informed about emerging trends and best practices to remain effective in their roles.
By developing these skills, network engineers can move beyond traditional operational tasks and take on more strategic responsibilities. Rather than being replaced by AI, they will lead the way in designing intelligent networks that drive business value.
Challenges in implementing AI for network engineering
Despite its potential, integrating AI into network engineering presents several challenges which organizations must address.
- Safety and oversight: While AI systems are robust, they are not infallible. Hallucinations or errors can lead to misconfigurations, security vulnerabilities, or unplanned downtime. To mitigate risks, engineers must validate AI outputs and maintain oversight.
- Regulatory compliance: AI-driven network management involves processing large volumes of data, making compliance with data protection regulations essential. Engineers should work closely with legal teams to ensure AI systems meet governance, security, and privacy requirements.
- Talent shortages: The demand for AI-savvy engineers exceeds supply, necessitating investment in upskilling programs that equip engineers with AI literacy, data analysis skills, and familiarity with AI-driven network platforms.
- Legacy infrastructure: Many organisations rely on outdated systems that are incompatible with AI-driven workflows. Upgrading to modern, adaptive architectures is essential but can be costly and disruptive.
Addressing these challenges requires a combination of technology investment, workforce development, and careful governance. AI adoption will not be seamless, but organisations that proactively manage these hurdles will gain a competitive edge.
Building a future-ready workforce
To prepare for the AI era, IT leaders must adopt a strategic approach that combines talent development, infrastructure modernisation, and cultural transformation.
Organisations should offer training on AI systems, data literacy, and advanced network management techniques.
Infrastructure modernisation is also likely to be required. Transitioning to flexible, software-defined architectures will enable dynamic optimisation and support for innovative applications.
Also, cultural transformation will be necessary. Encouraging experimentation and iterative learning fosters an environment where engineers can explore AI capabilities without fear of failure.
AI represents both a challenge and an opportunity for network engineering. By automating routine tasks and enhancing decision-making, it empowers engineers to focus on strategic initiatives.
The future of network engineering is not about replacing humans with AI but about enabling them to work smarter and more strategically. Enterprises that embrace this shift will be well-positioned to achieve new levels of agility, competitiveness, and innovation in an AI-driven world.